Understanding E-Commerce Data Analytics
E-commerce data analytics involves examining vast amounts of data collected from various digital touchpoints. The goal is to extract meaningful insights that can guide decision-making and strategic planning. Leveraging data analytics helps in understanding customer behaviors, identifying trends, and optimizing marketing efforts.
Key Components of E-Commerce Data Analytics
- Data Collection: Gathering data from sources like website interactions, social media, customer reviews, and purchase history.
- Data Processing: Cleaning and preparing data to ensure accuracy and consistency. This step involves removing duplicates, handling missing values, and standardizing formats.
- Data Analysis: Utilizing statistical methods and algorithms to uncover patterns and trends. Common techniques include descriptive analytics, predictive analytics, and prescriptive analytics.
- Data Visualization: Presenting data insights through charts, graphs, and dashboards. Effective visualization makes complex data comprehensible.
Common Techniques
- Descriptive Analytics: Summarizes past data to understand what happened. For example, tracking monthly sales revenue.
- Predictive Analytics: Uses historical data to forecast future outcomes. For instance, predicting next month’s sales based on previous trends.
- Prescriptive Analytics: Suggests actions based on data insights. An example is recommending promotional strategies to increase sales.
- Google Analytics: Provides insights into website traffic, user behavior, and conversion rates.
- Tableau: Allows for interactive data visualizations and dashboards.
- R and Python: Used for statistical analysis and data modeling.
- CRM Systems: Manage customer relationships and track interactions, providing valuable customer data.
Implementing e-commerce data analytics requires understanding these components, techniques, and tools. By doing so, businesses can make informed decisions that enhance customer experience and drive growth.
Essential Tools for E-Commerce Data Analytics
A wide variety of tools empower e-commerce data analytics. The right mix of these tools enables businesses to extract, process, and analyze data efficiently. Below are essential tools categorized into web analytics, customer relationship management, and business intelligence tools.
Web Analytics Tools
- Google Analytics: Google Analytics tracks website traffic, user behavior, and conversion rates. It enables me to understand customer journeys and optimize website performance.
- Adobe Analytics: Adobe Analytics offers web analytics solutions with robust segmentation and custom analytics capabilities. It helps me gain deeper insights into customer segments and engagement.
- Hotjar: Hotjar records user interactions and provides heatmaps, session recordings, and surveys. It lets me identify user pain points and improve the user experience.
Customer Relationship Management (CRM) Tools
- Salesforce: Salesforce manages customer data, tracks interactions, and automates sales processes. It allows me to tailor marketing efforts based on customer data insights.
- HubSpot: HubSpot offers a suite of CRM tools that facilitate marketing, sales, and customer service. It consolidates my customer interactions into one platform for seamless engagement.
- Zoho CRM: Zoho CRM tracks sales, automates routine tasks, and gives real-time insights. It supports me in maintaining strong relationships with customers through comprehensive data management.
- Tableau: Tableau visualizes complex data sets, allowing for intuitive data analysis and reporting. It lets me create interactive dashboards that facilitate informed decision-making.
- Microsoft Power BI: Microsoft Power BI combines data from various sources and provides powerful analytics and visualization tools. It enables me to share insights across teams easily.
- Looker: Looker offers data exploration and analytics, providing a holistic view of business performance. It allows me to uncover trends and derive actionable insights from the data.
By integrating these tools, businesses can enhance their data analytics capabilities, uncover valuable insights, and optimize their e-commerce operations.
Key Techniques in E-Commerce Data Analytics
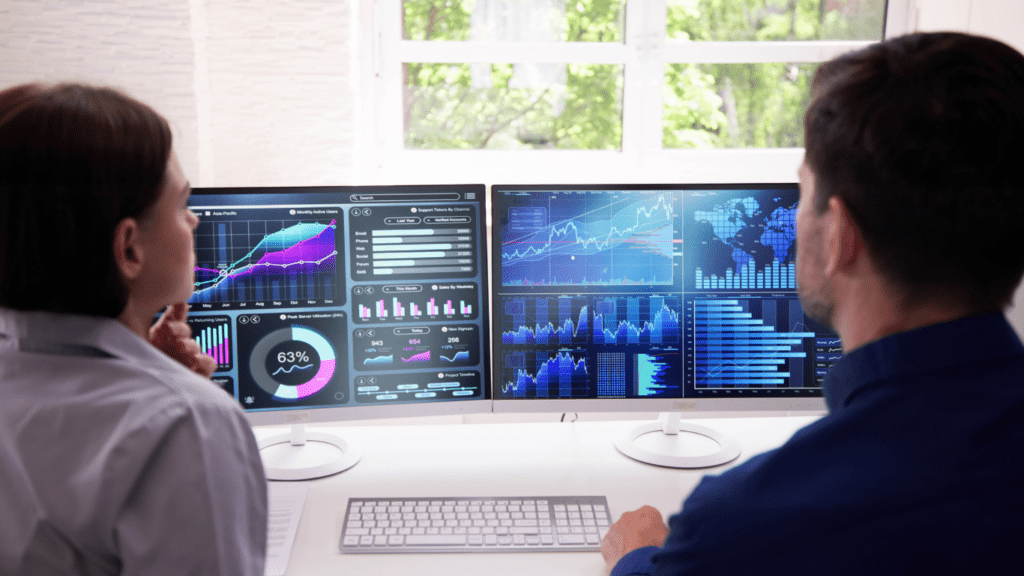
E-commerce businesses benefit from various analytics techniques that enhance customer understanding and decision-making. Among these, A/B testing, customer segmentation, and predictive analytics stand out.
A/B Testing
A/B testing compares two versions of a webpage or app to determine which performs better. I set up controlled experiments, presenting different versions to segments of visitors. Analyzing click-through and conversion rates helps identify the most effective elements. This iterative process optimizes user experiences and boosts sales. Optimizely and Google Optimize are popular tools for this technique.
Customer Segmentation
Customer segmentation groups users based on shared characteristics. I analyze demographic, behavioral, and transactional data to create targeted marketing strategies. Segmentation increases engagement and conversion rates by catering to specific needs. Parameters include location, purchase history, and behavior patterns. Tools like CRM systems (Salesforce, HubSpot) and analytics platforms (Tableau, Microsoft Power BI) assist in this process.
Predictive Analytics
Predictive analytics anticipates future trends by analyzing historical data. I utilize machine learning models to forecast customer behavior, sales, and inventory needs. Techniques include regression analysis, decision trees, and neural networks. This approach enables proactive strategies, enhancing inventory management and personalized marketing. R, Python, and specialized software like RapidMiner are essential tools for predictive analytics.
Benefits of E-Commerce Data Analytics
E-commerce data analytics provides critical insights that drive business growth. Analyzing data helps identify trends and patterns, enabling informed decisions.
Improved Customer Experience
Personalization becomes achievable by understanding customer behaviors and preferences. For example, using browsing history and purchase patterns, businesses can create tailored recommendations, improving customer satisfaction and retention.
Enhanced Marketing Strategies
Data analytics enables targeting specific customer segments more effectively. By analyzing demographics and purchase behaviors, marketing campaigns become more strategic and impactful. For example, personalized email campaigns yield higher open and conversion rates.
Efficient Inventory Management
Predictive analytics forecasts demand accurately. Businesses optimize stock levels, reducing excess inventory and minimizing stockouts. For instance, using historical sales data predicts seasonal demand spikes, ensuring adequate stock.
Increased Sales and Revenue
Identifying high-performing products and customer segments boosts sales. Data-driven insights enable upselling and cross-selling opportunities. For example, recommending complementary products during checkout increases average order value.
Better Decision-Making
Data visualization tools like Tableau and Power BI present complex data clearly, facilitating quick decision-making. Visual dashboards help track key performance indicators, like sales and customer acquisition costs, ensuring business goals are met.
Competitive Advantage
Companies leveraging data analytics uncover market trends and competitor strategies. Staying ahead by adapting to changes quickly ensures a competitive edge. For example, real-time analytics identify emerging market trends, allowing prompt strategy adjustments.
Cost Savings
Optimizing operations and marketing efforts through data analytics results in cost reductions. For instance, identifying inefficient marketing channels reallocates budget to more effective ones, maximizing ROI.
Risk Management
Data analytics identifies potential risks before they become issues. By analyzing customer feedback and market trends, businesses proactively address problems. For example, sentiment analysis on social media detects negative trends, enabling quick responses to mitigate damage.
Customer Retention
Understanding churn patterns allows businesses to develop retention strategies. Analyzing why customers leave helps implement measures to improve loyalty. For example, offering personalized discounts to at-risk customers increases retention rates.
Challenges and Limitations
E-commerce data analytics offers significant benefits but comes with challenges. One major issue is data quality. Inconsistent, incomplete, or inaccurate data can skew analysis results. It’s essential to implement robust data cleaning and validation processes to ensure reliability.
Another challenge is data integration. E-commerce platforms often utilize multiple systems such as CRM, ERP, and third-party marketing tools. Consolidating data from these systems into a unified view requires sophisticated integration tools and strategies.
Scalability is also a concern. As businesses grow, the volume of data increases. Analytics tools must handle large datasets efficiently to deliver timely insights without performance bottlenecks.
Privacy and security pose limitations. Handling personal and sensitive customer data necessitates strict compliance with regulations like GDPR and CCPA. Failure to protect this data can result in legal issues and loss of customer trust.
Interpreting data accurately requires expertise. Not all businesses have in-house data scientists or analysts. Misinterpreting analytics can lead to misguided strategies. Thus, investing in training or hiring skilled professionals is crucial.
Finally, the cost of analytics tools and infrastructure can be prohibitive, especially for small businesses. Implementing and maintaining advanced analytics requires significant investment. Cost-effective solutions and careful resource allocation become necessary for these enterprises.